Edge computing is a new computing layer located between the devices/embedded systems and the cloud, at the “edge of the network”. Edge nodes are connected and each one can perform computations and provide storage for several devices/embedded systems.
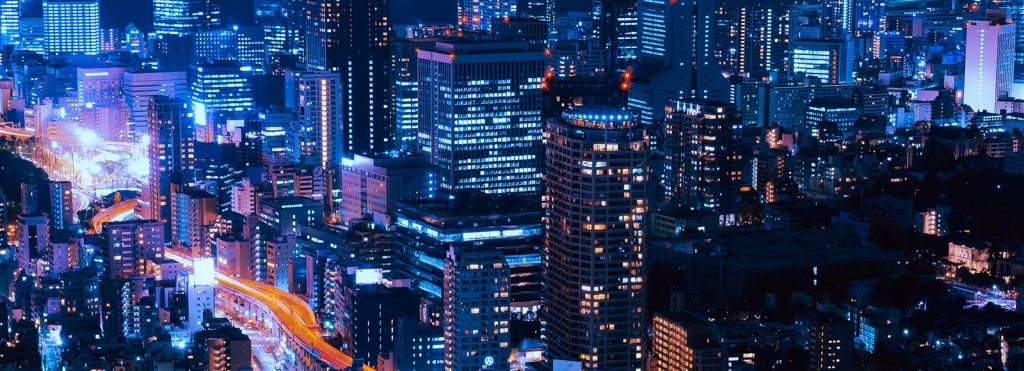
Addressing issues caused by the large amount of data generated by an ever increasing amount of connected smart devices and sensors, edge-based systems can provide low response times, relieve network capacity, and contribute to enhanced privacy. The technology has been made possible by AI, machine learning and and sensors used mainly by Cyber-Physical Systems and applications of the Internet of Things.
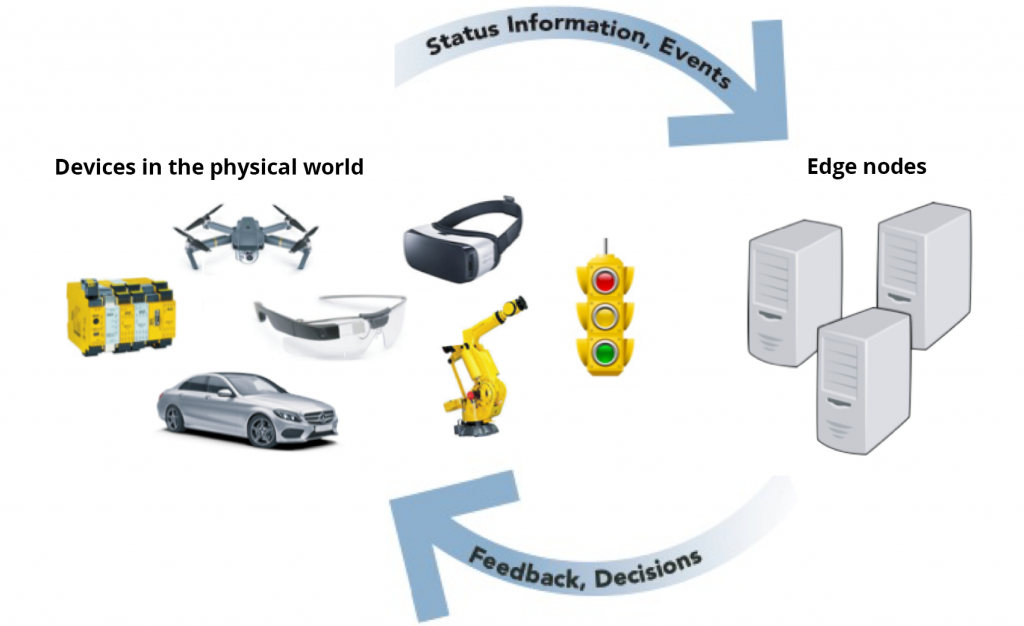
Benefits
One of the most significant societal trends is the accelerated digitalization of industries, including development processes as well as products and services. Performed in the right way, industrial digitalization leads to lower production costs, higher efficiencies as well as the realization of new use cases with significant value-adds.
These effects, costs savings as well as the introduction of new services, will have major impacts on value chains, and are therefore at the focus of virtually all industries globally. Nevertheless, digitalization comes also with major challenges. Its benefits increase with the amount of digitized objects and processes, which dramatically increase the amount of involved software and data volumes, raising the crucial question where the data will be processed and stored. Today’s architecture of large-scale IT infrastructures allows two possibilities: Either at the device level (i.e. embedded systems, mobile phones etc.) or at the cloud. However, both possibilities are insufficient.
Handling of data volumes
Handling the data volumes at the device level drives up device requirements for processing and storage. As this scales with the number of devices, the involved costs are unsustainable. Handling the data at the cloud – on the other hand – requires the transmission of the data volumes to remote compute centers, which is again not sustainable due to the associated costs for transmission. In addition, handling the data at the cloud diminishes or even prohibits the real-time interaction between the cyber domain and the physical objects due to the involved latencies.
Therefore, recently the academia and industry came to the realization that novel compute resources are required where data handling for digitalization can take place in a sustainable manner, i.e. cost-, energy- and resource-efficient. The key feature of these resources is that they are spatially close while still aggregating over larger sets of devices. The paradigm of spatially close computation is referred to as edge computing.
Cost reduction
Edge computing reduces costs on the side of the devices (less compute and storage is required) and overcomes the latency and bandwidth issues related to centralized cloud-based solutions. Due to these technical advantages, edge computing systems have a tremendous application potential with a projected global market in the order of tens of billions of dollars by 2022.
This market potential primarily arises due to three different application types enabled by edge computing: (1) Interactive human-in-the-loop applications; (2) Datadriven real-time analytics; and (3) Automated and collaborative cyber-physical systems (CPS). Interactive human-in-the-loop applications (like augmented reality and cognitive assistance) typically capture the motion and environment of humans, and provide perceptual feedback to humans with respect to task execution. They rely on edge computing systems primarily due to their low latency.
The same is true for collaborative CPS (e.g. cars at an intersection), where instead of the motion and environment of humans, the corresponding parameters of (semi)-autonomous systems are maintained at the edge, subsequently allowing efficient group coordination. In contrast, data-driven analytics (such as predictive maintenance) benefits from the bandwidth savings in comparison to cloud computing. The typical use case here is the real-time monitoring of machinery and equipment. If this is executed at the edge, complex analysis can be performed at much lower costs in comparison to the cloud due to the bandwidth savings.